Most meetings or conversation about quantifiable reliability improvement usually end up in a discussion of data and how bad it is. How do we navigate around this impasse?
It does seem as though most maintenance discussions, whether in formal meetings or in the corridor, include a frustrated restatement of the inadequacy of data. Why are we so pre-occupied with data? It’s because we believe that data holds the secrets to everything. Scientific discovery usually progresses via the analysis of experimental data. Why does the “scientific method” seem to evaporate at the doorstep of the maintenance department? You’ve asked yourself this question, doubtless, a thousand times.
This list divides data into two major categories, and a number of sub-categories.
- Age data (aka life cycle data, or event data)
- Data describing the beginning of a life cycle
- Data describing the ending of a life cycle.
- Ending by failure
- Functional failure
- Potential failure
- Ending by suspension
- Ending by failure
- Non-rejuvenating events
- Condition monitoring data
- CBM data from programs and instruments specifically set up to predict and mitigate failure consequences
- Process data from the control system collected in real time databases
- Internal variables reflect the degradation of equipment or process health (e.g. drifting of a temperature or pressure differential).
- External variables reflect the stresses imposed on the asset.
Age data and condition monitoring data are in reality two sides of the same coin. But they reside, often, in separate information systems. Consequently, maintenance engineers seldom visualize data from both sources together. Simultaneous analysis raises the exciting prospect that we may discover patterns in the condition data that correlate with, even predict, age data (failure) events.
Of the two major types of data, the age data gives us the most headaches. Why? It is because:
- We rely on a compilation of ambiguous “failure codes” on the work order to represent failure modes,
- We omit, from the work order the Event type of a failure mode instance (failure or suspension) required for reliability analysis.
LRCM solves both these problems. It matches a knowledge record with a work order. And, it provides the ending event type sub-categories, usually one of FF, PF, and S[1]. The combination of knowledge record and event type enables generation, by software, of an “events table”. Once in that form an unbiased sample may be extracted for analysis.
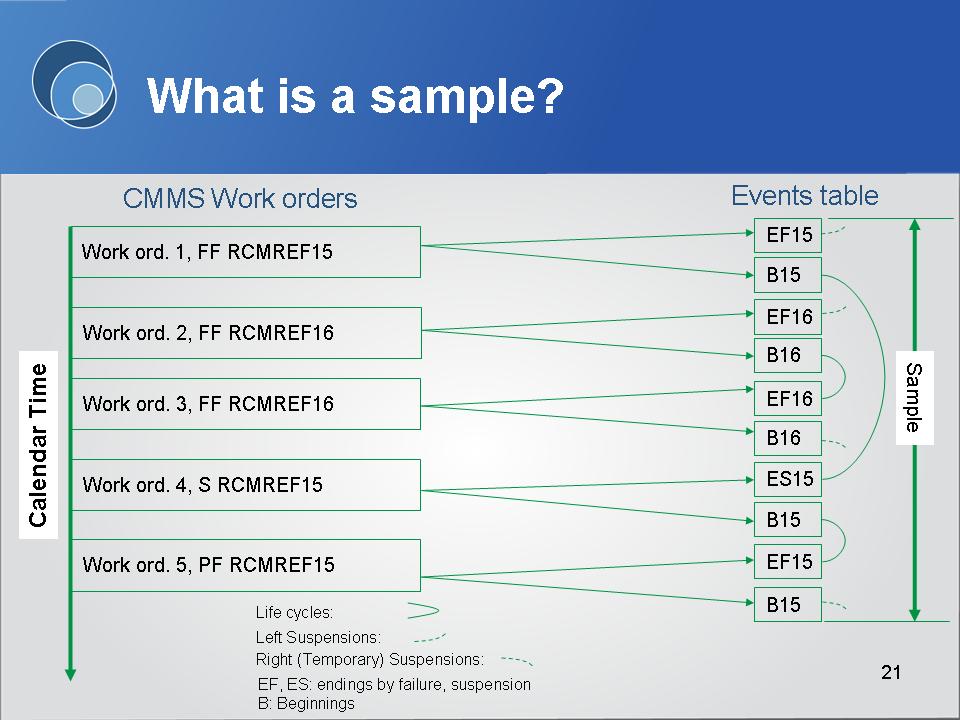
To generate a “Sample” for analysis, the EAM/CMMS work order records must be transformed into an Events table from which a sample may be generated for analysis and modeling.
The events table feeds well formed data to reliability analysis software, satisfying, finally, our voracious appetite for relevant data with which to improve maintenance policy.
© 2011 – 2014, Murray Wiseman. All rights reserved.
- [1]Functional Failure, Potential Failure, and Suspension respectively↩
- The data barrier to analysis (100%)
- Elevator description of LRCM (100%)
- How much data is required for RA? (100%)
- LRCM-EXAKT - a general solution (100%)
- The reliability data Catch 22 (100%)
- Take the EAM data health check (RANDOM - 100%)