Which, among limitless, maintenance related data is relevant to physical asset reliability improvement? Secondly, how do we transform that data into decision models for effective risk management? Finally, how do we continuously update those models for verifiable asset reliability improvement? These questions drive our relentless pursuit of new maintenance technologies. This article reports on Living RCM as a solution to the long standing problem of achieving physical asset reliability from data.
1. Introduction
Managers and maintenance engineers imagine a future where technology can offer a kind of “magic box” that collects and assesses relevant data, identifies recurring modes of failure, predicts the remnant lifetime of critical parts and recommends an optimal moment for intervention by a repair crew. Responding to this vision the maintenance technology industry has reasoned that the maintenance process should include certain activities and information sources, namely:
- Condition monitoring of vital equipment and systems,
- Failure prognostic algorithms with which to process relevant data, and
- Equipment failure and maintenance records (also known as “age data”)
Activities 1 and 2 have attained notable technical maturity over the years. Data acquisition systems, sensors and decision support software abound in the market. The missing element (item 3) has remained stubbornly elusive. The Enterprise Asset Management (EAM) system often fails to satisfy the reliability analyst’s need for consistent, accurate, and complete age data. Unfortunately, realistic prognostic algorithms (item 2) for practical maintenance decision making depend on adequate input from item 3.
Age data is a record of events where a part has either failed or was renewed preventively in order to preempt its failure. Disappointingly, the EAM process often fails to satisfy the requirement for accurate and complete age data. Carbones del Cerrejón, a mining operation in Colombia, discovered the reliability data “gap” when its reliability engineers attempted to apply prognostic decision modeling algorithms to fleet maintenance planning. They failed to achieve the required level of Condition Based Maintenance (CBM) predictive performance.
Standard deviation is a quantitative measure of confidence in the Remaining Useful Life Estimate (RULE) of a component. The lower the standard deviation the better the CBM tactic meets its performance objective. The standard deviation is calculated to report the performance of a CBM model or policy. Carbones del Cerrejón’s reliability analysts applied Proportional Hazard Model (PHM) Analysis [1]to their CBM and EAM databases and found that the latter contained failure and replacement data that was inconsistent, inaccurate, or incomplete rendering prognostic performance too low for practical CBM decisions. Upon investigation the reliability engineers uncovered the reason for the inadequacy. Technicians lacked an unambiguous, simple method by which to record observed instances of a Failure Mode and their ending Event types (either Failure, Potential Failure, or Suspension). This age data is a prerequisite for effective reliability analysis and CBM decision modeling.
To resolve the problem Carbones del Cerrejón set out to implement a “Living” RCM (LRCM) process that would guarantee perfect transcription of a technician’s observations following execution of each work order. Accurate age data allows Cerrejon’s analysts to construct prognostic models by correlating instances of failure (as determined by age data) with condition monitoring data patterns preceding the failure event. For this type of analysis a data sample must differentiate between failures and suspensions. A suspension is a renewal of a Failure Mode (e.g. a part or component) for reasons other than failure. Pattern finding procedures need to “know” whether a given ending event was actually due to failure. Mislabeling a suspension as a failure would mislead the algorithm and result in poor predictive performance. The LRCM process ensures that each work order will represent a valid data point in a statistical sample. A sample is a collection of Failure Mode life cycles that includes their ending age and state. The age and state (failure or suspension) of a Failure Mode at its life ending constitute its “age data”. This article describes the benefits that can be attained by applying Reliability Analysis (RA) procedures such as Proportional Hazard Modeling (PHM) to historical records comprising data of sufficient quality so as to be analyzable. “Analyzable data” consists of age data that is at least 90% accurate. Such accuracy can be achieved through the MESH LRCM work order documentation process.
2. The LRCM Process
The MESH LRCM knowledge update and work order data strategies ensure analysis quality data for practical maintenance decision making. These strategies were implemented and tested in Carbones del Cerrejón to meet the following requirements:
- Accurate and complete age data recorded with minimal effort as necessary for deploying verifiable optimal prognostic decision models.
- Dynamic update of the Knowledge Base as contained in RCM, FMEA databases, and represented in the EAM failure related catalogs in order to ensure that:
- Work order pick lists reflect unambiguously the range of reality as observed by a technician during work order execution, and that
- The maintenance plan will be updated regularly so that it responds optimally to up-to-date knowledge regarding the probability and severity of the effects of each reasonably likely failure mode.
The LRCM process fulfills the stated corporate requirement that physical asset maintenance performance improve continuously. Good equipment reliability, safety, and productivity depend on the quality and timeliness of data. LRCM, by making the right data available, ensures competent staff, good prognostic decision models, and a process of continuous improvement. A discussion of these targets follows.
3. The right information
The framework of information required for maintenance decision support has four main groups:
- The RCM Knowledge Base: A data structure describing the functions, failures, failure modes, effects, consequences and mitigation activities with respect to a physical asset.
- Age data: Also known as “life data”, it measures the asset’s expended “effort” resulting in accumulated damage over time. Age can be measured in calendar time, but more realistically by hours of operation, production units, consumed energy, or some other measure proportional to accumulated stress on the asset. Age data must accurately and consistently record:
- The failure mode: The part that failed and, optionally, the mechanism of deterioration and underlying cause.
- The beginning event: The event that identifies the beginning of the life of a part or Failure Mode.
- The ending event: The age of the part and the way in which its life ended, either by:
- Failure,
- Potential Failure, or by
- Suspension
- Condition Monitoring Data: Includes process data measurement, visual inspection data, and sensor data. These data may be catagorized as “internal” or “external” variables. Internal variables reflect actual internal damage. External variables record operational stresses that, eventually, result in internal damage.
- Business factors: Business data, with respect to a given failure mode, quantify the penalty for failure relative to the cost of prevention.
Of the four information groups the most challenging is the reliable capture of age data. MESH LRCM captures analyzable data by dynamically linking work orders with the Knowledge Base in a new type of work order user interface.
4. Qualified personnel:
The MESH LRCM solution is primarily a human-oriented process that monitors performance in metrics that each employee can, himself, impact. One such performance index measures the rate of improvement in the reliability knowledge base. This indicator reports the count of Reliability Centered Maintenance (RCM) elements added or updated via the LRCM knowledge feedback procedure integrated with the work order.
A culture that emphasizes leading indicators, for example the quality of collective knowledge or the accuracy of work order documentation will ultimately impact the lagging performance metrics related more directly to profitability or mission readiness. Those involved in the living reliability process (technicians, supervisors, analysts, superintendents, managers, supervisors, and health and safety specialists) appreciate the recognition in the LRCM database of their participation in their organization’s success. Simple procedures implemented quickly “on-the-job” enable a short learning curve so that these metrics are observable within weeks from LRCM implementation.
5. Good decision models
A decision model is a rule or algorithm that maintenance personnel use to decide upon a course of action in a certain recognizable recurring situation. For example, given a component’s current age and condition monitoring data one of these decisions will be taken:
- Intervene in the operation of equipment and perform a specified maintenance action immediately,
- Perform an intrusive maintenance inspection within a prescribed time period, or
- Defer the maintenance decision until the next data observation.
The procedure or model used to take the decision is know as a “policy”. A maintenance decision policy also must be verifiable over time. The manager must monitor the policy’s performance, How “good” are the decisions flowing from a given policy? Does the policy need to be changed or improved? To facilitate the management challenge maintenance decision models should report on their own performance. How well did the model meet equipment availability and cost objectives by optimizing the balance between preventive and proactive maintenance. MESH LRCM ensures that the work order system delivers the data needed for managing maintenance policies.
6. Continuous Improvement:
Continuous improvement in maintenance requires that the RCM knowledge base align ever closer with reality. Day-to-day decision policy and the failure mitigation plan must adapt to new observations in the field. The initial RCM analysis was a “first approximation” having captured the analysts’ incomplete recollection of past equipment failures. Operating contexts change, often in subtle ways. Technology advances. Field experience is a voyage of constant discovery. A Living RCM process responds to new information as technicians document their findings upon executing work orders. The LRCM feedback mechanism exposes any divergence between observation and currently documented failure modes, effects, and consequences. Additionally, the LRCM work order user interface displays reliability (MTBF) at the granularity of the failure mode, highlighting the efficacy of current mitigation strategies. Technicians and engineers, so informed, initiate recommendations for updating the current strategy by using the non-intrusive LRCM feedback mechanism. Thus, in a natural process, technicians and analysts identify and address recurrent failure modes impacting performance. The diagram illustrates LRCM inserted in the maintenance process.
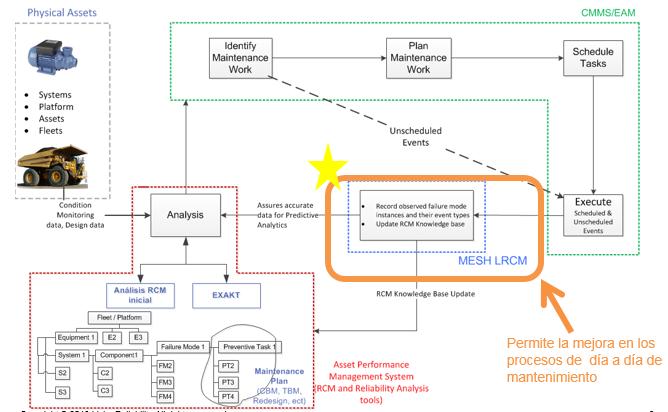
MESH LRCM integrated with the maintenance process ensures the correct data for analysis and decision modeling
MESH LRCM is a modular technology solution. The following sections describe the functionality from the viewpoints of each member of the maintenance team. The Manager and Superintendents implement strategies that support of high level (lagging) performance metrics. On the other hand, supervisors, analysts and technicians focus on day-to-day operational effectiveness as reflected in leading metrics.
7. The maintenance manager
What was the goal in introducing MESH LRCM into your organization? I was inspired by the idea of linking actual cost and uptime performance to strategies and policies at ground level that we could easily monitor and improve.
What has been the impact of MESH LRCM so far? MESH LRCM monitors “low level” indicators. By “low level” I mean measurements of actual behaviors which, logically, bear indirectly on the high level metrics of interest to our shareholders, such as equipment up-time, operational cost, and profitability.
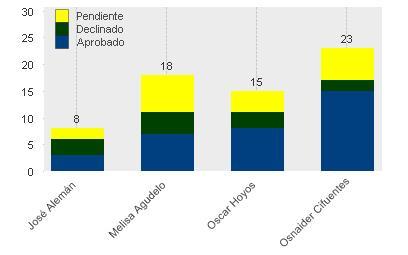
Figure 2 KPI low : Suggestions to the RCM Knowledge Base made by technicians during the work order completion process
One such low level “leading” performance index quantifies the rate of improvement in the quality of data recorded on the work order. Instinctively, we know accurate, complete, relevant data will translate to better decisions and better performance. Also, MESH reports the number and types of approved updates to the RCM Knowledge Base. Employees themselves directly control these leading indicators. These metrics reflect desirable working habits. The holy grail of management is to discover a traceable connection between the low and high level indicators. It is the low or leading indicators that propel the daily management of maintenance. Figures 2 and 3 show low-level indicators for a particular equipment type or fleet.
In Figure 3 the high peaks of the first few months following the start of the project correspond to the surge of employee suggestions for improving the reliability knowledge base. Eventually the number diminishes as the knowledge base reflects a closer understanding of the reality of equipment failure behavior.
8. The superintendent
How does Living RCM assist your role as superintendent? The MESH LRCM system allows us more control over the performance of the fleet. This is because:
- Information coming into the EAM corresponds to what was actually found prior to executing a work order.
- The work order information recorded through LRCM is relevant, concise, complete and accurate. The LRCM procedure encourages consistent terminology. Now I place greater value on the reports and summaries I receive. I am able to take decisions with greater confidence.
- My analysts spend far less time cleaning data and more time analyzing information and incorporating it into the decision process.
- Additionally, we are consolidating knowledge from experienced staff and contractors in an organized and structured way. This ensures a continuous increase in technical skills.
- I can attest that we have reduced the number of events of certain recurrent failures due to the focus on the failure instance count in the knowledge tree displayed in the work order user interface.
- From the analyst’s reports we have statistical evidence at this early stage that we improved our CBM prognostic performance.
- An issue that we have been aware of for some time is the lack of standards for differentiating between failures and suspensions in the huge variety of failure modes experienced in the fleet. The MESH image galleries associated with each failure mode assist technicians and supervisors in establishing consistent definitions of failure, potential failure, and suspension.
- Previously the “Effects” field in RCM was given little attention. Now it has become the enabler of improved communication among those involved in the process of maintenance
9. The supervisor
What changes have you perceived since MESH has been implemented with regard to your daily interactions? I would say that communication is quicker and less strained? We are adopting a single language with which to define maintenance concepts such as failure, potential failure, and suspensions relative to each failure mode. RCM has always been for us the accepted terminology. We recognize its simplicity but at the same time its preciseness, for example regarding the consequences of failure. It is helpful when technicians, supervisors and analysts converse in terms of functions , failures, failure modes , effects and consequences. This allows us to identify and record consistently what what was found, what was done, which parts failed, and which parts were preemptively renewed. My role as supervisor can be more efficiently dedicated to providing my technicians with whatever they need to get equipment back up with the least amount of delay. What’s interesting is that they themselves contribute to the RCM knowledge base in RCM elemental language and they gain the pride that their individual knowledge contributions are recorded and recognized.
10. The technician
Has LRCM contributed to your work? Yes, LRCM has improved our efficiency in a number of ways, for example:
- We are working more closely with the Analysts and management. We better understand each other’s goals and see more clearly the benefits shared
by all parties. That is, we consolidate our respective knowledge. We are the ones who capture information about the equipment’s failure modes when that information is most clear and complete – right after doing the job. This increased accuracy does not add to our work load. But it does make our job more interesting and satisfying.
- Where before it was difficult and frustrating to select the correct failure mode in the work order now it is quick and we are certain that the information we provide is correct.
- The MESH system supports and encourages updating of the knowledge base by the persons who execute maintenance tasks – that is we, the technicians. Now the supervisors, planners and engineers benefit from our detailed knowledge and observations. By using the MESH feedback system we impact the maintenance plan. This is empowering and satisfying.
- MESH has eliminated guesswork when selecting the Failure Mode(s) on completion of a work order. When we make our selection we do so with the benefit of all known information about that Failure Mode its Effects, Consequences and current mitigation strategy. All this information appears instantly just by right clicking on that node in the RCM tree as displayed right on the work order. If that knowledge does not match our observations we quickly initiate a “feedback” on the spot. The Analyst responsible for knowledge base of the fleet or equipment type acts upon our feedback. Within a few days we witness our contribution to the knowledge base. We can even list in a report all knowledge contributions that a given employee originated.
- When necessary to describe a failure or suspension we can illustrate our knowledge suggestions with photos or sketches. We can upload the images directly into the knowledge feedback system integrated with the work order user interface.
11. The reliability analyst
How has Living RCM impacted the analytic and decision process?
The idea behind Condition Based Maintenance (CBM) decision modeling is really quite simple. We compile Condition Monitoring (CM) data and concurrent age data for a given equipment. Then we apply an algorithm that seeks out patterns in the CM data that correlate with (predict) actual failures as reported in the age data. Obviously then, if the age data in the EAM is incorrect so too will be our decision models. Fortunately, as illustrated below, we have a way of using statistics to measure confidence in decision model performance.
The great improvement is in the confidence related to model based decisions. When work orders inconsistently report failure modes or mislabel Suspensions as Failures, analytic models lose their usefulness. The diagram below illustrates the relationship between prognostic performance and input data quality.
The diagram of the Remaining Useful Life estimate (RULE) illustrates that the usefulness of reliability analysis depends on the quality of the EAM data.[2] The mean of the conditional density function curve is the RULE reported from the processing of the data. The spread or width of the curve is a measure (standard deviation) of confidence, which relates strongly to EAM work order data quality.
What improvements in the information for analysis?
By monitoring completed work orders we have seen a significant improvement in data from the field and shop.
What decision support does the predictive maintenance program give?
For a given component, part, or failure mode, it gives us an unambiguous recommendation, a Remaining Useful Life Estimate (RULE), and a confidence measure.
The top image is a summary exception report listing components and failure modes, their respective prognostic, and optimal repair recommendation. The analyst can drill down and receive detailed graphs such as those at the bottom of the image. These graphs show the history of the weighted sum of the variables found to be significant to failure, the failure probability, the remaining useful life, and measure of confidence (standard deviation).
12. The HSE (Health, Safety, Environment) manager
How has LRCM impacted Health, Safety, and Environmental responsibility
HSE performance is a business goal no less critical than is profitability. Poor performance in HSE can dramatically reduce profitability. As in any objective, we must encourage work habits and behavior that lead to the desired HSE performance metrics. LRCM offers a proactive methodology for recognizing HSE risk as a natural activity integrated with day-to-day work procedures. Accidents and environmental excursion are always consequences of a failure mode. LRCM is a methodology that encourages periodic revisits to a failure mode and reassess its potential risk. We repeatedly pose the question, “Do the mitigating activities match the severity and probability of occurance of the failure mode?”. Hence LRCM provides us with a simple integrated process for HSE management and continuous improvement.
Should an accident or disaster or near miss occur, the RCM knowledge trail within the MESH software allows us to know the state of our knowledge just prior to the incident. Had the failure mode been identified? Were the effects and consequences anticipated? Was the mitigating activity adequate? What was lacking in the analysis that permitted the incident to occur?
© 2015 – 2021, Murray Wiseman. All rights reserved.
- [1]A method that tests each condition variable’s correlation with actual failure events in order to determine its influence on the probability of failure. (Examples). ↩
- [2]For more information on the effect of misidentifying suspensions see the article “Defeating CBM“↩
[…] CM decision tools, to be effective, must be supported with good EAM data.[3] The Living RCM methodology enables EAM users to ensure analysis quality work order data from shop and the field technicians. More information on LRCM is given in the article Achieving reliability from data. […]